CRO Glossary
User Testing
User testing is a research-driven evaluation process where real users interact with a website, app, product, or service to assess its usability, functionality, and overall user experience. By observing how participants complete tasks in realistic conditions, businesses can identify pain points, navigation challenges, and areas for improvement before a full-scale launch.
For meaningful insights, user testers should be given minimal guidance, allowing them to explore the interface naturally. This ensures that the system is intuitive, user-friendly, and accessible to individuals unfamiliar with it. Effective user testing helps businesses refine design, enhance usability, and ultimately create a more seamless and satisfying experience for their audience.
What is User Testing?
As I’ve already defined, user testing is the process of evaluating a product’s interface and functions by observing real users as they perform specific tasks. This approach provides invaluable insights into the natural behaviors and preferences of users.
For example, during user tests for a new e-commerce website, participants might be asked to find and purchase a specific item. Observing how easily they can navigate the site, find the product, and complete the purchase helps identify any usability issues or areas of confusion.
By understanding these interactions, developers can make informed decisions to enhance the usability, functionality, and overall user experience of the product. This iterative process of testing and refining is essential for creating a product that not only meets but exceeds user expectations.
Why Is User Testing Important?
User testing is crucial for identifying and resolving usability issues before a product, app, or website is launched. By observing users in real-world scenarios, businesses can uncover problems that might not be evident during internal testing.
Key benefits of user tests include:
- Identifying Usability Issues: Discovering problems that users face while interacting with the product, app, or website.
- Highlighting Errors and Gaps: Uncovering missing requirements or gaps in the software that could hinder performance.
- Gathering Real User Feedback: Obtaining direct input from users to make informed design and functionality decisions.
- Enhancing User Satisfaction: Improving the overall experience to meet and exceed user expectations.
- Increasing Conversion Rates: Refining the product to boost user retention and conversion rates.
Conducting these tests can be done manually or using automated tools, both of which provide critical data to refine the product, app, or website.
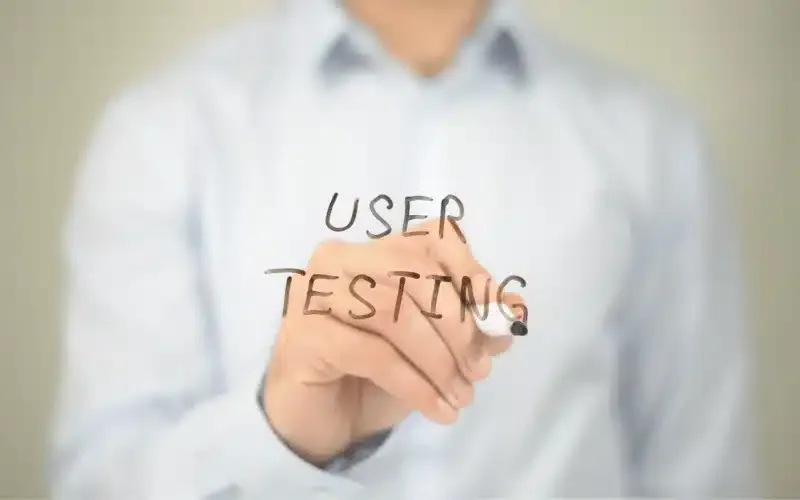
Types of User Testing
User testing can be categorized into several types, each focusing on different aspects of the user experience. Understanding and employing the right type of customer testing can significantly enhance the usability and effectiveness of a product, app, or website. Below are some common types of user tests with detailed examples:
Usability Testing (UX Research)
What does usability testing mean? We can define this concept as the evaluation of a web page, electronic interface, or e-commerce site by real users to assess its efficiency and ease of use. The goal is to ensure that users can navigate the site effortlessly, find what they are looking for, and achieve their goals without frustration.
In other words, when users visit an e-commerce site, and they cannot find what they want or encounter errors, it is not due to their lack of technical skills. Instead, it is the fault of the online store for not being user-friendly.
Website usability is a mandatory requirement for an e-commerce site. An online store that does not fulfill its purpose of selling products due to poor usability cannot be considered effective. Usability issues can lead to user frustration, abandoned carts, and ultimately, business failure. Therefore, ensuring a smooth and intuitive user experience is critical for success.
Key Metrics in Usability Testing:
In usability testing, various aspects are measured to help developers pinpoint usability issues and areas for improvement, ensuring that the product meets user needs and expectations.
- Eye-Tracking
- Heat Maps
- Click-Through Maps
- User Journey Mapping
Example of Usability Testing:
Imagine you are testing an e-commerce website that sells clothing. The usability test involves real users who are asked to find and purchase a specific item, such as a pair of shoes. During this process, the following observations are made:
- Navigation: Users navigate through the site, using categories and filters to locate the desired product. If users struggle with filtering options, it may indicate that the filters are not intuitive or comprehensive enough.
- Product Selection: Users view product details, check sizes, and add the item to their cart. Observing how users interact with product pages can reveal if important information is easy to find.
- Checkout Process: Users proceed to checkout, where they enter their shipping and payment information. If users encounter issues with form fields, payment options, or understanding the total cost, these are recorded.
Surveys
Another type of user testing is represented by surveys, which help you to obtain user feedback about your product from your customers. It’s one of the easiest ways to obtain data from your customers because customers can complete surveys from any device and location.
It’s recommended to appeal to customer satisfaction surveys if you want to receive, in a short time, a lot of responses from your customers regarding your product. The information gathered will help your UX designer to have clear indications in the design process and on information architecture to deliver a perfect user journey for your customers.
Key Metrics in Surveys:
- Response Rate: The percentage of users who complete the survey out of the total number invited.
- Net Promoter Score (NPS): Measures customer loyalty by asking how likely users are to recommend the product to others.
- Customer Satisfaction Score (CSAT): Measures user satisfaction with specific aspects of the product.
- Open-Ended Feedback: Provides qualitative insights from user comments and suggestions.
Example of Survey Usage:
Imagine you have launched a new feature in your mobile app and want to gather user feedback. You create a survey asking users about their experience with the new feature. The survey includes questions about ease of use, functionality, and overall satisfaction. Users are invited to complete the survey through an in-app notification.
If users report difficulty in using the new feature, this indicates a need for improved user interface design.
High satisfaction scores can validate the success of the new feature, while low scores highlight areas for improvement.
A/B Testing
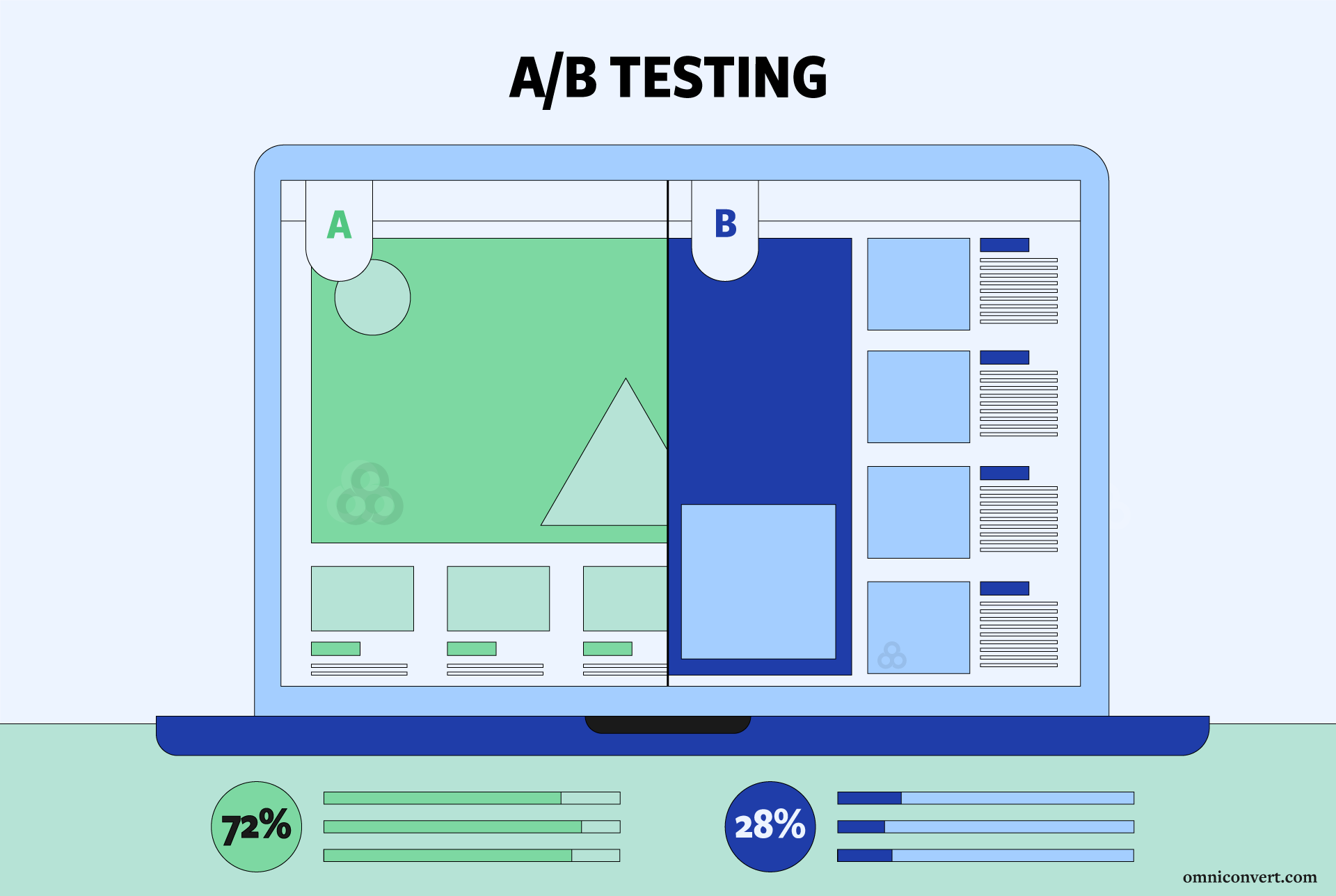
This type of testing is one of the most efficient and fast ways to increase the number of conversions. A/B testing involves sending two different variants of an email, webpage, or other content to different groups of users to compare their effectiveness. It is important to remember that each group must receive only one variant of the email, not both, and they must be sent on the same day and at the same time.
Then, track customer reactions and determine which variant has aroused the reactions that help you meet the proposed goals. A/B testing helps you learn a lot about your site visitors and the type of content they respond best to, allowing for data-driven improvements.
Key Metrics in A/B Testing:
- Conversion Rate: Measures the percentage of users who complete the desired action (e.g., subscribing to a newsletter, making a purchase) for each variant.
- Click-Through Rate (CTR): Tracks the percentage of users who click on a link or button in each variant.
- Bounce Rate: Measures the percentage of users who leave the site after viewing only one page, helping to identify which variant keeps users engaged.
- Time on Page: Indicates how long users stay on a page, providing insights into which variant holds their attention longer.
Example of A/B Testing:
Imagine you are conducting A/B testing to increase the number of newsletter subscriptions on your website. You decide to test the following elements:
- Variant A: A registration form with fewer fields (only name and email) and a prominent call-to-action button that says “Subscribe Now.”
- Variant B: A registration form with additional fields (name, email, age, and interests) and a call-to-action button that says “Join Our Community.”
These forms are shown to different groups of website visitors at the same time.
If Variant A results in a higher subscription rate, it suggests that users prefer a simpler form with fewer fields. A higher CTR for the call-to-action button in Variant A indicates that the phrase “Subscribe Now” is more effective. A lower bounce rate for Variant A suggests that users are more likely to complete the form when it is short and straightforward. If users spend less time on the page with Variant A but still convert, it indicates that a quick, easy process is preferable.
By analyzing these metrics, you can conclude that a shorter registration form with a clear call-to-action is more effective in increasing newsletter subscriptions. This insight can then be used to optimize the registration process, leading to higher conversion rates and a better user experience.
Focus Group
The focus group is a qualitative research technique that involves gathering a small group of 8-10 participants for a guided discussion on a specific topic, typically lasting 1-2 hours. This method is invaluable for obtaining detailed insights into the motivations and behaviors of target demographics.
This technique can be used in various fields, such as:
- Testing messages, products, advertisements, etc.
- Identifying perceptions about a product, organization, service, or concept
- Evaluation/testing of advertising and promotional campaigns
- Identification of the profile of a target group
- Identifying the characteristics of a brand (brand image) and positioning it among competing brands on the market
- Identifying the decision-making mechanisms that underlie the choice between several alternatives
- Identification of attitudes toward a product, an idea or a problem
- Identifying the set of values and aspirations of a target segment
- Drafting an advertising campaign, and marketing strategy
- Establishing the strengths and weaknesses of a concept, program, product/brand, etc.
Sometimes focus-group research is also a preamble to quantitative research because by identifying behavioral tendencies, it is easier to compose the questionnaire for quantitative research.
Key Metrics in Focus Groups:
- Engagement Rate: Measures the level of participant interaction and contribution during the discussion.
- Topic Exploration: Tracks the depth of discussion around key topics or questions.
- Group Consensus: Evaluates the degree of agreement or divergence on specific issues among participants.
Example of Focus Group Usage:
Imagine you are developing a new line of skincare products. To understand consumer preferences and perceptions, you conduct a focus group with individuals who regularly use skincare products. During the session:
- Participants discuss their current skincare routines, preferred ingredients, and their expectations from skincare products.
- They evaluate prototypes of your new products, providing feedback on packaging, scent, texture, and effectiveness.
- Insights from the focus group help refine product formulations, adjust marketing messaging, and optimize the product lineup to better meet consumer needs and preferences.
This qualitative feedback provides deeper insights into message clarity, visual impact, brand engagement, and competitive positioning. These insights guide iterative improvements to ensure the campaign resonates effectively with the audience, enhancing engagement and conversion rates.
Beta Testing
The Beta version represents the final stage of software development before release to end-users, undergoing testing to identify and resolve issues. Websites, operating systems, and various applications can enter the Beta testing phase, which may be open for public testing or restricted to specific groups. Open Beta testing allows real-world usage scenarios, aiding developers in identifying and rectifying potential issues.
Beta testing aims to finalize performance evaluations and address any remaining errors that could impact functionality. It provides valuable feedback, reports errors, and gives suggestions that help enhance features and refine the product before its official release.
Key Metrics in Beta Testing:
- Bug Reports: Number of bugs reported per tester or per feature.
- Feedback Volume: Quantity of feedback received from beta testers.
- Feedback Quality: Evaluation of feedback based on its relevance and actionable insights.
- Completion Rate: Percentage of testers who complete the beta testing phase.
- Error Rate: Frequency of errors encountered by testers.
Example of Beta Testing:
Imagine you’re developing a new mobile app for scheduling tasks. During beta testing, you release the app to a group of 100 users who regularly provide feedback. Metrics show a high bug report rate in the first week, prompting your team to focus on fixing critical issues like crashes and slow performance. As beta testers continue to use the app, they suggest improvements to the user interface, leading to adjustments in design elements and feature enhancements before the official launch.
How to Do User Testing?
In order to gain as many relevant insights as possible from user tests, thorough preparation is required. For example, it should be clearly defined right from the beginning to whom the end product is aimed. For this purpose, it is advisable to develop personas and scenarios in advance. Testing tools help the project team throughout the implementation to gain a shared understanding of the end user’s essential needs.
1. Define a Goal
The form and extent to which a user-test is performed depend on the objective being pursued.
The approach varies significantly based on whether:
- An existing page is reviewed due to a planned redesign, aiming to identify usability issues and gather insights for improvement.
- A new function is tested for usability, focusing on evaluating its effectiveness and user-friendliness.
- Decision-makers need to be convinced with the help of a test, showcasing user preferences and highlighting areas of concern.
The starting point of each user test is, therefore, primarily the determination of the objective of the investigation and what exactly should be achieved. Based on this, further steps of the user-testing are derived and decisions made.
2. Prepare the Test Object
Lo-Fidelity Prototypes
Lo-Fidelity prototypes are basic, simplified versions of a product used at the very beginning of a project. They are primarily used to validate an initial concept or idea. These prototypes are stripped of high-fidelity elements such as detailed design and visual effects. By keeping the prototype simple, the feedback obtained from test participants focuses solely on core functionality and is not influenced by aesthetics.
Hi-Fidelity Prototypes
Hi-Fidelity prototypes are detailed, advanced versions that closely resemble the final product. These include finished websites, apps, or precise visual designs. They provide comprehensive feedback on both content and visual design, helping to refine the user experience before the final release.
For both types of prototypes, it’s important to simulate the desired user experience as realistically as possible. For instance, a Lo-Fidelity prototype might use a simple illustration of a mobile phone interface, while a Hi-Fidelity prototype should be tested on an actual mobile device to ensure an accurate user experience.
3. Select the Test Method
There is a diversity of test methods, and each expert has his personal preferences. The choice of the appropriate method should depend primarily on the maturity of the prototype being tested.
For the purpose of this discussion, we will focus on moderated in-house user testing.
Moderated in-house customer testing involves a facilitator guiding participants through tasks in a controlled environment. The facilitator observes and interacts with the users, asking questions and exploring their behaviors and thoughts. This approach is great for understanding user motivations, preferences, and issues.
Choosing the right test method based on your prototype and goals ensures the insights gathered are useful and actionable. Moderated in-house testing helps you deeply understand how users interact with your product, leading to better design decisions and a better user experience.
4. Write a Test Script
A test script is a detailed plan that guides the moderator through the user test process. It ensures consistency and helps gather comparable results from each participant.
A typical test script consists of a warmup, a body, and a cooldown. It takes between 5 to 10 tasks and usually takes between 30 and 60 minutes to complete. To get the most out of your time and get comparable results, a well-structured test script is needed. It serves as a guide for the moderator and will not be given to the test person.
Each task in the script comes with a hypothesis (why you are testing this task) and a goal (what you hope to learn). Before starting the tasks, explain the context to the participant with a scenario. This scenario helps them understand their role and the relevance of the tasks. After setting the scene, the participant can then proceed with the tasks and questions as guided by the script.
5. Recruit Test Subjects
You’ll need to locate and recruit test subjects to complete your user evaluation. Regardless of your favorite way of software testing (user feedback, eye tracking, mouse movements), consumer testing requires real clients, actual members of your intended audience, and those who match your client personas to take the test. This permits you to find outcomes and data from the men and women who matter most; the customers who buy, use, and promote your goods. People Interacting with your product are best in user research.
Recruiting the right subjects for the test can be time-consuming and should not be underestimated. To keep the bounce rate low, recruitment should be made promptly on the test date (at most 3-4 days in advance). Always recruit more participants than needed to account for no-shows. Those who undertake the recruitment themselves should plan sufficient time to write to the test persons, to screen them through a survey, and to provide appropriate letters before the test.
How to Find Users for Testing:
- Customer Lists: Use your existing customer database to identify and reach out to potential participants who fit your target personas.
- Social Media: Leverage your social media platforms to recruit participants by posting about the opportunity and its benefits.
- User Testing Platforms: Utilize online platforms like UserTesting, UserZoom, or Respondent.io to find and recruit test participants.
- Website Pop-Ups: Implement pop-ups on your website inviting visitors to participate in user testing.
- Email Campaigns: Send out targeted emails to your subscribers inviting them to participate in the testing process.
- Incentives: Offer incentives such as gift cards, discounts, or exclusive access to new features to encourage participation.
Selection Criteria:
To get valuable results, ensure your test subjects match your actual target group (personas). A balanced mix of subjects is important to avoid biased results. Avoid using team members, employees, family, or friends, as they may have prior knowledge or biases that affect the results. Random test subjects should also be avoided as they may not represent your target audience accurately.
Number of Subjects:
One of the most discussed topics, when it comes to uncovering usability issues, is the right number of subjects. Studies show that 5 test persons are enough to identify the most critical issues in the product. However, if statistical relevance is important, you may need more participants.
Scheduling:
It is recommended that the schedule for each user test is not too close to each other. A short break of about 30 minutes after each round leaves room for the unforeseen and allows a short exchange among the participants to discuss the findings.
To ensure a smooth process and to prevent subjects from appearing late, in the wrong place, or not at all because of missing information, provide all participants with the address, a contact name, and an emergency number early enough. Short memory on the morning before the test prevents the subjects from forgetting their appointment.
6. Prepare the Site and Infrastructure
The space you choose for the test should be quiet and spread a pleasant atmosphere. The subject should feel as comfortable as possible. If several people want to follow the test, it makes sense to add a second room, if possible, not within earshot. The audience can then follow the progress of the test on a screen without the test person feeling disturbed.
Ensure all necessary equipment is in place and functioning correctly. This includes computers, mobile devices, cameras for recording sessions, and any specific software required for the test. Test all equipment beforehand to avoid technical issues during the session.
7. Carry out a Test Run
To be certain that nothing goes wrong during the actual test, a test run with an uninvolved person should be done before that. Thus, it can be determined whether the planned time frame can be met, the technical setup works, and the instructions to the test persons are understandable and consistent.
8. Evaluation and Analysis
The evaluation of the data should take place as soon as possible after the execution; otherwise, one runs the risk that important details are forgotten. If possible, all involved experts (facilitator and observer) should write down their first personal assessments individually and independently. If the assessments are compared afterward, the result is a more neutral evaluation. Also, the likelihood is less than a problem that will be overlooked.
Be aware that rough prototypes or unrealistic testing conditions can cause usability problems that might not occur under real-world conditions. It’s important to distinguish between issues caused by the prototype or test setup and actual user problems. Ensure that the evaluation considers the limitations of the testing conditions. Problems arising solely from the prototype’s roughness or unrealistic conditions should be noted separately to avoid conflating real user issues with those caused by the test environment.
Conduct a detailed analysis of the feedback, focusing on identifying usability issues, user pain points, and areas for improvement. Categorize the problems based on severity and frequency to prioritize solutions. By following these steps, the evaluation and analysis process becomes thorough, accurate, and actionable, leading to meaningful improvements in the product’s usability and overall user experience.
User Testing Metrics
User testing metrics are essential for evaluating the effectiveness and usability of a product. They provide quantitative data that helps understand user behavior, identify pain points, and improve the overall user experience. Here are some key user test metrics:
Task Success Rate
This metric measures the percentage of tasks that users complete successfully without any assistance. A high task success rate indicates that the product is intuitive and easy to use, while a low success rate highlights areas that need improvement.
Time on Task
This measures the amount of time users take to complete a specific task. Shorter times generally indicate a more efficient and user-friendly design. However, it’s important to balance speed with accuracy and satisfaction.
Error Rate
This metric tracks the number of errors users make while performing tasks. High error rates can indicate confusing interfaces or problematic features. Analyzing these errors helps in identifying and fixing usability issues.
System Usability Scale (SUS)
The SUS is a standardized questionnaire that provides a quick assessment of the product’s usability. It consists of ten questions and results in a score out of 100. Higher scores indicate better usability.
Customer Satisfaction (CSAT)
CSAT measures how satisfied users are with the product. This can be gathered through post-task surveys or feedback forms. High satisfaction scores indicate a positive user experience.
Net Promoter Score (NPS)
NPS measures the likelihood of users recommending the product to others. It is based on a single question survey and provides insights into overall user loyalty and satisfaction.
User Testing vs. Usability Testing
User Testing: This refers to observing the emotions, responses, and behaviors of a customer from the moment they start using your product until they stop. It encompasses the entire user journey and focuses on understanding the overall experience, including the satisfaction, engagement, and emotional reactions of the user.
Usability Testing: This is a specific method within user tests that focuses on how easily and effectively a customer can use your product to accomplish a specific goal. Usability testing evaluates the functionality, efficiency, and user-friendliness of the product but does not cover the entire user experience. It is more about identifying specific usability issues and improving the product’s interface and design.
User experience testing: User experience testing involves collecting both qualitative and quantitative data from users to enhance their overall experience. This process aims to gather insights that can lead to improvements in product design, functionality, and user satisfaction. By focusing on both the emotional and functional aspects of user interaction, user experience testing provides a comprehensive view of how well the product meets user needs.
FAQs
Is User Testing Easy?
User testing can be complex and time-consuming, requiring careful planning and execution. The process typically involves recruiting participants, designing and conducting tests, analyzing the results, and making changes to the product or service based on the feedback received. User testing can be challenging because it requires a deep understanding of the user’s perspective and the ability to identify and address usability issues and other problems that may not be immediately apparent.
Is the user testing legitimate?
Yes, user testing is entirely legitimate. It provides valuable insights that help improve product design, functionality, and overall user satisfaction, ensuring that the product meets the needs and expectations of real users.