The Power of Natural Language Processing (NLP) in Survey Results Interpretation

Article last updated:
Article first published:
NLP gives computers the ability to understand and interpret human language. See how NLP can help you find the needle in your data haystack.
Each day, approximately 328.77 million terabytes of data flood the digital landscape - an amount almost impossible to comprehend.
To put it into perspective, 328.77 million terabytes would be equivalent to about 5.48 billion years of continuous video watching (assuming each minute of video takes about 1 gigabyte of space.)
Looking at this enormous scale of information one can find online, it’s easy to understand why data analysts are finding it increasingly challenging to keep up.
Fortunately, the same analysts now have a game-changing tool at their disposal, helping them make sense of the data in ways that were once unimaginable: Natural Language Processing (NLP).
NLP gives computers the ability to understand and interpret human language, allowing them to sift through mountains of text and extract meaningful insights with lightning speed and precision.
Let’s explore the topic and see how NLP can help you find the needle in your data haystack.
What is Natual Language Processing (NLP)
Natural Language Processing (NLP) is a field of AI focused on enabling computers to understand, interpret, and generate human language.
As tech evolved, so has NLP - around since the 1950s, it progressed from rule-based systems to statistical methods and machine learning.
NLP works by breaking down human language into words, phrases, and sentences and analyzing their relationships.
Key NLP tasks include:
- Tokenization: Breaking down text into individual words or tokens.
- Part-of-Speech Tagging: Identifying the grammatical parts of speech (e.g., nouns, verbs, adjectives) for each word in a sentence.
- Named Entity Recognition: Identifying and classifying named entities such as names of people, organizations, and locations in text.
- Syntax Parsing: Analyzing the grammatical structure of sentences to understand the relationships between words.
- Semantic Analysis: Extracting the meaning and intent behind sentences, including sentiment analysis and topic modeling.
NLP has revolutionized various applications. To name a few, think virtual assistants, chatbots, language translation, sentiment analysis, and information extraction.
In a nutshell, NLP enhances human-computer interaction and data processing capabilities.
Using NLP for Open-ended Survey Questions
Any eCommerce business that truly cares about its customers will treat open-ended questions as a non negotiable in their strategy.
These questions allow customers to express themselves freely, providing rich and nuanced feedback.
However, depending on the sample size, it can become a challenge to manually analyze a great volume of unstructured text data, then identify patterns and themes.
NLP automates and streamlines the process.
Its algorithms can parse through large volumes of text data, identify key phrases, sentiments, and topics, and categorize responses based on themes and sentiments.
This automation significantly reduces the time and effort required for manual analysis, allowing you to extract actionable insights more efficiently.
For example, let's consider an eCommerce business conducting a customer satisfaction survey.
One of the open-ended questions asks customers to describe their experience with the website's checkout process.
Without NLP, analyzing thousands of responses would be time-consuming and labor-intensive.
However, by leveraging NLP, the business can quickly identify common themes such as usability issues, payment problems, or shipping concerns.
They can also pinpoint the overall sentiment towards the checkout process, identifying areas for improvement and addressing customer pain points more effectively.
In a nutshell, NLP empowers you to better understand customer needs, improve the user experience, and drive overall customer satisfaction and loyalty.
NLP and NPS
First things first, let's talk about NPS – Net Promoter Score.
If you're not familiar, NPS is a simple yet powerful metric used by businesses to measure customer loyalty and satisfaction.
It's based on one straightforward question: "On a scale of 0 to 10, how likely are you to recommend our product/service to a friend or colleague?"
Customers' responses are categorized into Promoters (score 9-10), Passives (score 7-8), and Detractors (score 0-6).
The NPS provides a clear indicator of customer sentiment and helps identify areas that might hurt your reputation and your customer loyalty efforts.
However, while NPS scores offer valuable insights into overall customer satisfaction, they often leave businesses wondering why customers feel the way they do.
That's where NLP comes in.
Through analyzing the verbatim comments that accompany NPS scores, NLP uncovers the "why" behind customers' ratings.
It sifts through all feedback data to identify recurring themes, sentiments, and specific pain points mentioned by customers.
With this deeper understanding, you can quickly spot areas for improvement with precision, whether it's enhancing product features, improving customer service, or addressing common concerns.
For example, let's say a company receives a high NPS score but notices a pattern of comments mentioning slow response times from customer support.
Without NLP, these insights might go unnoticed, or be discovered too late - leading to missed opportunities to fix the customer experience.
With NLP, businesses can spot these trends early on and take proactive steps to address them, ultimately giving customers what they need and driving business growth through customer loyalty.
Using NLP For Sentiment Analysis
Sentiment analysis, also called opinion mining, is an NLP tool helping you figure out if data carries a positive, negative, or neutral tone.
Businesses often use sentiment analysis to keep tabs on how customers feel about their brand and get a sense of what customers really want.
Sentiment Analysis and Survey Interpretation
As people increasingly express their thoughts and emotions openly, sentiment analysis rises as a powerful tool for monitoring and understanding sentiments across various data sources.
Sentiment analysis proves versatile across various survey types, be it quantitative or qualitative.
It also helps assess customer support interactions, empowering us to understand customer emotions and opinions.
Monitoring customer sentiment on a regular basis highlights any shifts in NPS scores or sentiment regarding specific business facets.
In other words, sentiment analysis provides immediate visibility into NLP fluctuations, allowing a granular understanding of the ever-changing customer experience, going beyond mere statistical figures.
The advantages of sentiment analysis are manifold:
- Managing Data Volume: Sentiment analysis streamlines the processing of vast amounts of unstructured data, sparing businesses from the arduous task of manual sorting.
- Real-Time Insights: Sentiment analysis enables the identification of critical issues in real-time, such as burgeoning social media crises or impending customer churn, facilitating swift action.
- Consistency in Analysis: Centralized sentiment analysis systems ensure consistent criteria application across all data, enhancing accuracy and insights.
Sentiment Analysis Tools and Methods
The first step in sentiment analysis is gathering relevant reviews and mentions from various places.
This includes customer comments on the company website, social media, and interactions with customer support. Sometimes, partnerships with data providers can also enrich the feedback.
Then, you have to tag words and phrases with sentiments like positive, neutral, or negative is crucial. It's more reliable when multiple people do this to ensure accuracy.
Before analysis, data needs cleaning.
This means getting rid of noise, unnecessary information, and common words that don't carry sentiment, making the analysis more accurate.
To make sense of text data, words and phrases need to be turned into numbers. So it’s easier for computer programs to understand and analyze the text.
Skilled data scientists train models using prepared datasets.
They split the data into parts for training and testing to ensure the models can accurately detect and classify sentiment.
There are many tools and APIs available for sentiment analysis, such as InMoment, Clarabridge, Azure AI Language, Google Cloud Natural Language API, and IBM Watson NLP.
Sentiment Analysis in Action
In Brazil, federal spending skyrocketed by 156% from 2007 to 2015, while satisfaction with public services steadily declined.
Concerned about this concerning trend, the Urban Planning Department turned to McKinsey for help in prioritizing user experience, or "citizen journeys," in service delivery.
This citizen-focused approach to governance has given rise to what we now call Smart Cities.
McKinsey developed City Voices, a tool that conducts citizen surveys covering more than 150 metrics and uses sentiment analysis to help leaders understand how constituents live and what they need, thus informing public policy decisions more effectively.
Through this tool, the Brazilian government identified urgent needs—like improving the safety of the bus system—and tackled them as top priorities.
If such initiatives can succeed on a national scale, just imagine the possibilities for your company.
Take Your Survey Results Interpretation to The Next Level
Survey interpretation can be tricky. One common challenge is gaining meaningful insights from the data. Traditional methods often provide only surface-level understanding, missing deeper sentiments and trends hidden within the responses.
Human bias is another pitfall. Interpretation can be influenced by subjective perspectives, leading to inaccurate conclusions and misguided decisions.
Moreover, the sheer volume of data can overwhelm analysts, making it difficult to extract relevant insights efficiently.
To overcome these challenges, it's essential to define clear objectives for survey analysis. Having a clear goal guides the interpretation process and ensures focus.
Using diverse analytical techniques is also key. Combining quantitative and qualitative analysis methods provides a holistic view of the data, revealing both numerical trends and underlying sentiments.
Cross-validation is crucial for ensuring the accuracy of survey findings. Comparing results with other data sources or methodologies validates the insights, enhancing their reliability.
Integrating NLP tools into survey systems offers a solution to these challenges.
NLP algorithms can analyze unstructured survey responses, extracting sentiment, themes, and trends that might otherwise go unnoticed.
Automating survey analysis with NLP streamlines the interpretation process, saving time and resources. By eliminating human bias, NLP tools provide more objective insights into customer sentiments and preferences.
Now, the most effective way to make the most of NLP processing in your business is by integrating a software suite designed to simplify complex data into actionable insights.
At the time of writing this article, we're in the Beta Phase for the Customer Experience module within Omniconvert's Reveal, making use of AI-powered Natural Language Processing.
Explore how our Customer Intelligence platform, Reveal, transforms raw data into customer-centered insights.
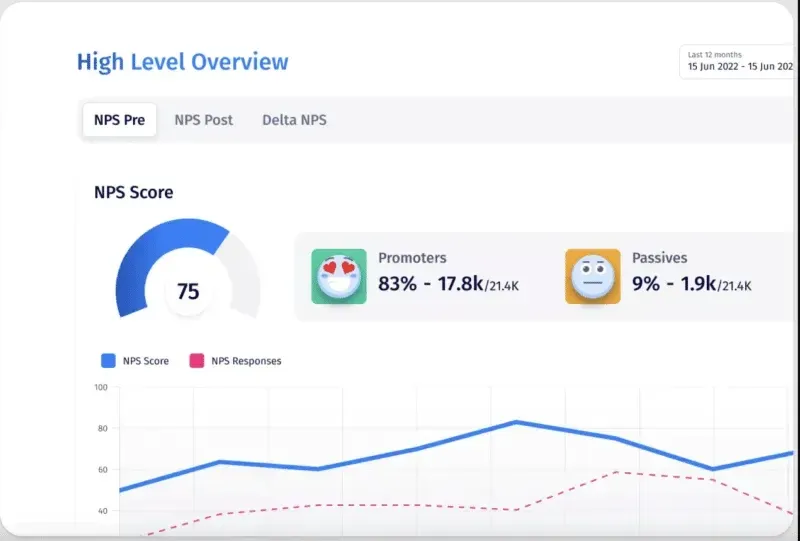
Discover how it turns data into inspiration, inspiration into action, and action into results!
Wrap Up
As you can see, NLP delves deep into customer feedback, revealing insights that traditional methods may miss.
It streamlines analysis, guards against bias, and empowers data-driven decision-making.
Ultimately, NLP is a game-changer, transforming interpretation, offering businesses a clearer understanding of customer sentiments and needs, and paving the way for innovation and growth.
Don't miss out on its potential in understanding your customers better and driving business growth!
If you liked this article, make it shine on your page :)